- Akgun, S., & Krajcik, J. (in press). Artificial intelligence as the growing actor in education: Addressing the issues of power and ethics of AI in K-12 STEM classrooms. In X. Zhai & J. Krajcik (Eds.), Uses of Artificial Intelligence in STEM Education (pp. xxx-xxx). Oxford University Press.
- Bradford, L. (in press). Use of machine learning to score teacher observations. In X. Zhai & J. Krajcik (Eds.), Uses of Artificial Intelligence in STEM Education (pp. xxx-xxx). Oxford University Press.
- He, P., Shin, N., Zhai, X., & Krajcik, J. (in press). Guiding teacher use of artificial intelligence-based knowledge-in-use assessment to improve instructional decisions: A conceptual framework. In X. Zhai & J. Krajcik (Eds.), Uses of Artificial Intelligence in STEM Education (pp. xxx-xxx). Oxford University Press.
- He, P. Shin, N. Kaldaras L., & Krajcik, J. (in press). Integrating artificial intelligence into learning progression-based learning systems to support student knowledge-in-use: Opportunities and challenges. In Jin, H., Yan, D., & Krajcik, J. Handbook of Research in Science Learning Progressions.
- Kaldaras, L., Akaeze, H., & Krajcik, J. (2021). Developing and validating Next Generation Science Standards-aligned learning progression to track three‐dimensional learning of electrical interactions in high school physical science. Journal of Research in Science Teaching, 58(4), 589-618.
- Kaldaras, L. (2020). Developing and validating NGSS-aligned 3D learning progression for electrical interactions in the context of 9th grade physical science curriculum. Michigan State University.
- Kaldaras L, Yoshida NR., & Haudek KC (2022) Rubric development for AI-enabled scoring of three-dimensional constructed-response assessment aligned to NGSS learning progression. Front. Educ. 7:983055. doi: 10.3389/feduc.2022.983055
- Kaldaras, L., Li, T., Haudek, K., & Krajcik, J. (accepted). NGSS learning progression-based scoring of scientific models. Paper submitted to the 2024 Annual Meeting of the American Educational Research Association (AERA), Philadelphia, PA.
- Kaldaras, L., Li, T., Haudek, K., & Krajcik, J. (accepted). Rubric development for AI scoring of NGSS learning progression-based scientific models to support individual opportunity to learn. Paper submitted to the 2024 annual conference of National Association of Research in Science Teaching (NARST), Denver, CO.
- Li, T., Liu, F., & Krajcik, J. (2023) Automatically assess elementary students’ hand-drawn scientific models using deep learning of Artificial Intelligence. Proceedings of the Annual meeting of the International Society of the Learning Sciences (ISLS).
- Li, T., Kaldaras, L., Haudek, K., & Krajcik, J. (accepted). Using AI to evaluate multi-modal formative assessments in physical science. Paper submitted to the 2024 Annual Meeting of the American Educational Research Association (AERA), Philadelphia, PA.
- Li, T., Kaldaras, L., Haudek, K., & Krajcik, J. (accepted). Utilizing deep learning AI to evaluate scientific models: overcoming the challenges. Paper submitted to the 2024 annual conference of National Association of Research in Science Teaching (NARST), Denver, CO.
- Shiroda, M., Doherty, J., & Haudek, K. C. (in press). Exploring attributes of successful machine learning assessments for scoring of undergraduate constructed response assessment items. In X. Zhai & J. Krajcik (Eds.), Uses of Artificial Intelligence in STEM Education (pp. xxx-xxx). Oxford University Press.
- Wang, H., Li, T., Haudek, K., Royse, E., Manzanares, M., Adams, S., Horne, L., & Romulo, C. (2023). Is ChatGPT a threat to formative assessment in college-level science? An analysis of linguistic and content-level features to classify response types. Proceedings of the 4th International Conference of Artificial Intelligence in Educational Technology (AIET).
- Zhai, X., He, P., & Krajcik, J. (2022). Applying machine learning to automatically assess scientific models. Journal of Research in Science Teaching, 59(10), 1765–1794. https://doi.org/10.1002/tea.21773.
- Zhai, X., & Krajcik, J. (in press). Pseudo artificial intelligence bias. In X. Zhai & J. Krajcik (Eds.), Uses of Artificial Intelligence in STEM Education (pp. xxx-xxx). Oxford University Press
- Zhai, X., & Krajcik, J. (Eds.). (in press). Uses of Artificial Intelligence in STEM Education. Oxford University Press.
Research
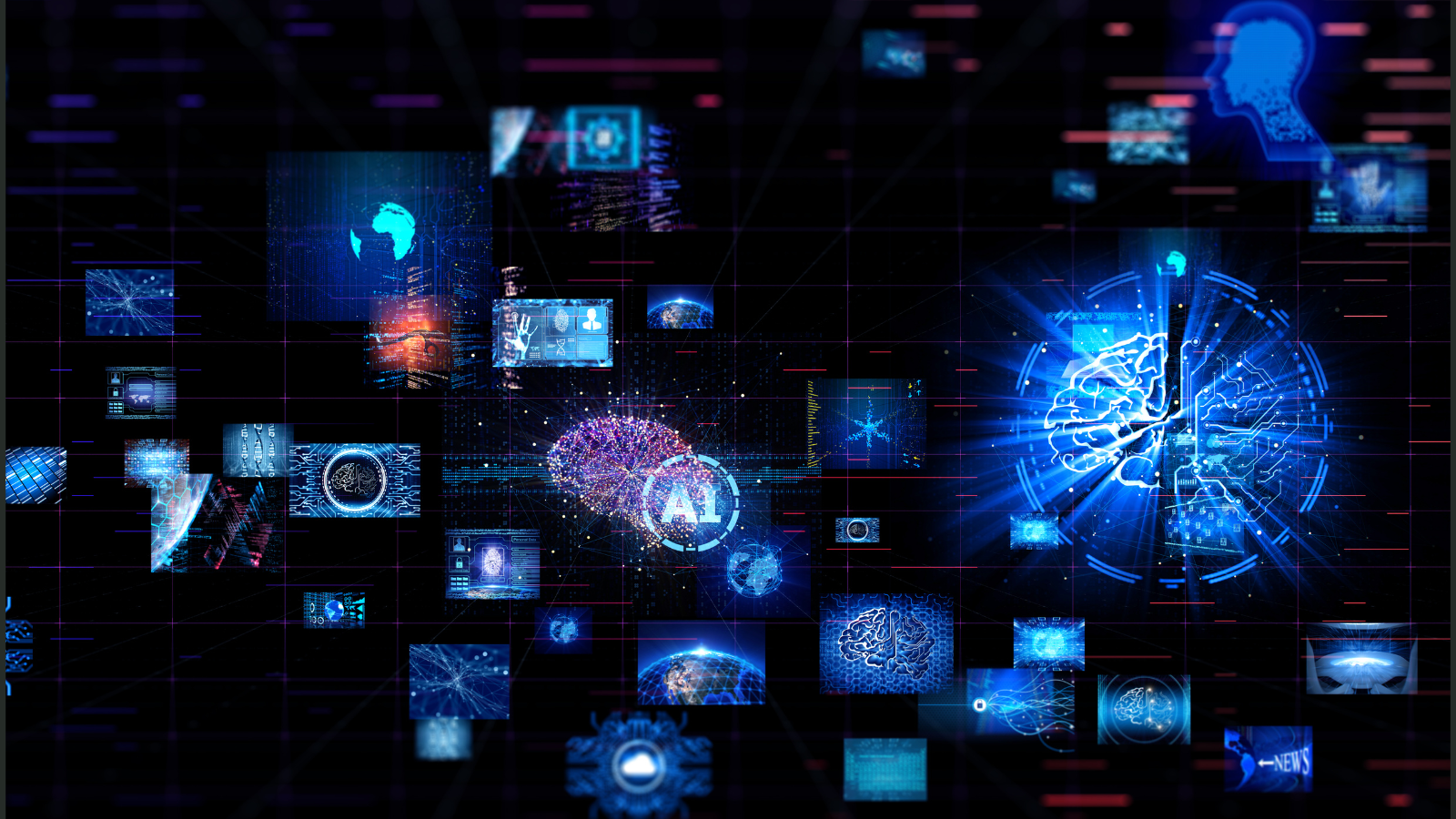
The Center for Research in Education and Emerging Technologies explores and pushes forward the use of artificial intelligence in all aspects of education. Today's students need opportunities to become knowledgeable about AI and its capabilities, and AI and other emerging technologies will provide them with new tools to help solve the multitude of problems facing the world today.
-
-
Subjective-Objective-Assessment Plan Notes Using Large Language Models
-
Empowering Future Teachers with Generative AI Education: Understanding Applications, Risks, and Limits
-
Auto Feedback 3DLP:Evaluating Effects of Automatic Feedback Aligned to a Learning Progression to Promote Knowledge-in-Use
-
Scaffolding Computational Thinking
-
Supporting Instructional Decision Making (PASTA)